Lies, Damned Lies, and Statistics AI
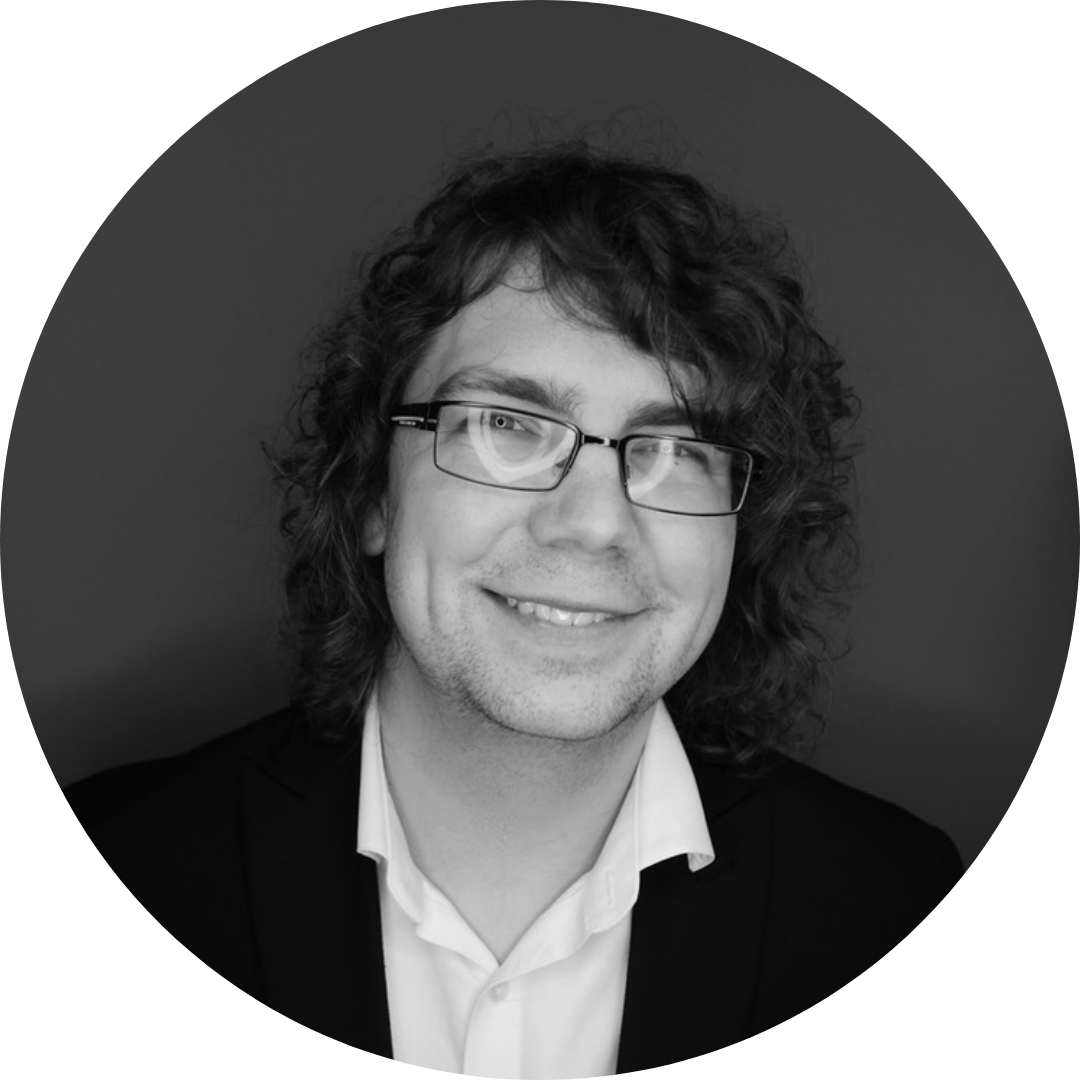
Matt Heys
Senior VP, Artificial Intelligence & Neural Genesis
We are all inherently biased individuals. We all harbour deep-seated, problematic views formed from the sum of our experiences and the insidious impact of wider discriminating societal ideologies and the hateful whims of media moguls. We all suck basically, and part of the human condition is to constantly try and break free of the negative and unhelpful thought patterns we’ve been conditioned into adopting as our own. For example: I hate cucumber – It’s a tasteless, watery, vegetable that doesn’t deserve the right to take the place of avocado maki in a sushi selection. Ok, so that’s a bit tame but I’m not exactly going to reel off a bunch of overtly racist, sexist or homophobic views and get myself CANCELLED™ am I? Also, I’m none of those things. This is all the proverbial, royal ‘we’ – i.e. ‘you’ all suck – ‘I’ am great.
Amazing start to a blog, right? Insult your audience, check ✅.
I’ve established the premise that humans are terrible and a scourge on societal harmony, BUT, at least now, thanks to the information age, we have a Monopoly get-out-of-jail-free card in the form of completely ‘objective’ artificial intelligence!
EXCEPT, no, we don’t.
Well, yes and no. There’s no malicious intent when it comes to machine learning models because, well, there’s no such concept as intent in machine learning models. At the end of the day, it’s all just maths (feel free to drop the ‘s’ if you’re American – it’s incorrect but I’ll allow it), algorithms, and probabilities – and whilst I may somewhat subscribe to a fatalistic view of existence, I still believe humans have the ability to ‘make decisions’; maths is deterministic. Mathematicians and physicists: Don’t come at me with your imaginary numbers and quantum realms, thanks.
So, AI alone cannot scan through a list of CVs and decide to reject candidates because it thinks their names sound ‘too ethnic’, they’ve mentioned a same-sex relationship, or they reference being in a wheelchair basketball team. AI could be instructed to do that, and without any form of moderation (a hot topic amongst the creators of foundational models), it may carry out the task – but it won’t just make those judgement calls on its own.
However, I stress the word ‘intent’ in all of this; maths cannot have intent. That doesn’t mean that machine learning models are free of any bias though. Unless an equation is mysteriously unearthed in the ruins of some ancient temple to the gods – most of our understanding of the world comes from observation vs. theory. Einstein was a clever dude, but he didn’t just magically say E=mc² and it became fact. In fact, the fact is, that scientists don’t really deal in facts. Theories exist for as long as they fit with observation and other theoretical models. When I was doing my degree in Physics, Astrophysics and Cosmology, we couldn’t reference papers from before 1998 due to the discovery that the expansion of the universe was accelerating – invalidating the accepted stance that a coefficient in Einstein’s Theory of General Relativity, the cosmologic constant, should be set to zero.
Sorry, got a bit physics-y there. The point I’m trying to make is that the way we apply maths is usually based on observation – and this is essentially how machine learning models are created. We pass in a large set of data, we observe the patterns in that data, and we create equations/models based on those observed patterns/interactions. For example, if you plot the number of deaths vs. age at time of death, you’ll notice an upwards trend – i.e. people tend to be older when they die, which matches with our expectations, so we can validate it. A basic model trained on this data will suggest the same thing because it’s just applying trends that it sees in the data and making predictions based on these. (1)
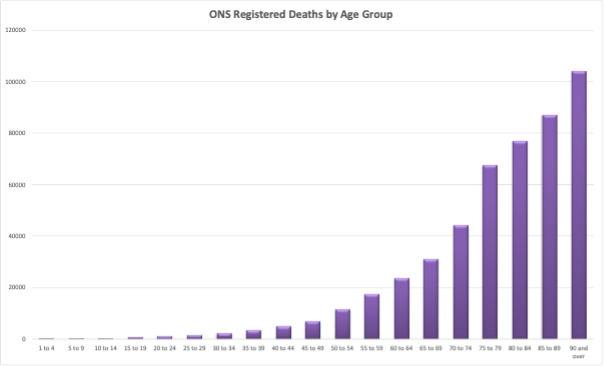
The problem comes when the data itself exposes problematic biases that exist in the real world. For example, the incarceration rate in the US is, on average, 6 times higher for black people than white people (2), which is widely understood to not be a reflection of criminality of different racial groups but of a fundamentally racist justice system. If we were to train machine learning models on this data and replace judges with cyborg adjudicators, making use of the outputs of these algorithms to determine the guilt of the accused, then a lot of innocent black people would be going to jail. However…this is already the case, so the cyborgs would just be continuing an unjust system.
Part of the solution is to identify where bias exists in data and then assess how the outputs of a model are to be used. The risk of maternal death is almost three times higher among women from Black ethnic minority backgrounds compared with White women (3). If we use this to help create targeted interventions for higher risk ethnic minority groups, then we’re acknowledging the bias and using it to try and change the underlying issue. If, however, we incorporate ethnicity into predicting whether a death was expected or not (i.e. the NHS has existing measures, Hospital Standardised Mortality Ratio (HSMR) and Summary Hospital-level Mortality Indicator (SHMI) – to my knowledge, these don’t currently include ethnicity), we could be ignoring many maternal deaths as ‘expected’ for people of colour. The data and model outputs remain the same in both cases – the underlying bias hasn’t changed – but it’s how we’re using the outputs that makes the difference.
So far, the examples I’ve suggested have been theoretical, at least in as far as I’ve described them. I’m not sure how long I’ve got before I’m murdered in my sleep by a rouge cyborg adjudicator but when that happens, avenge me dear reader. Avenge me.
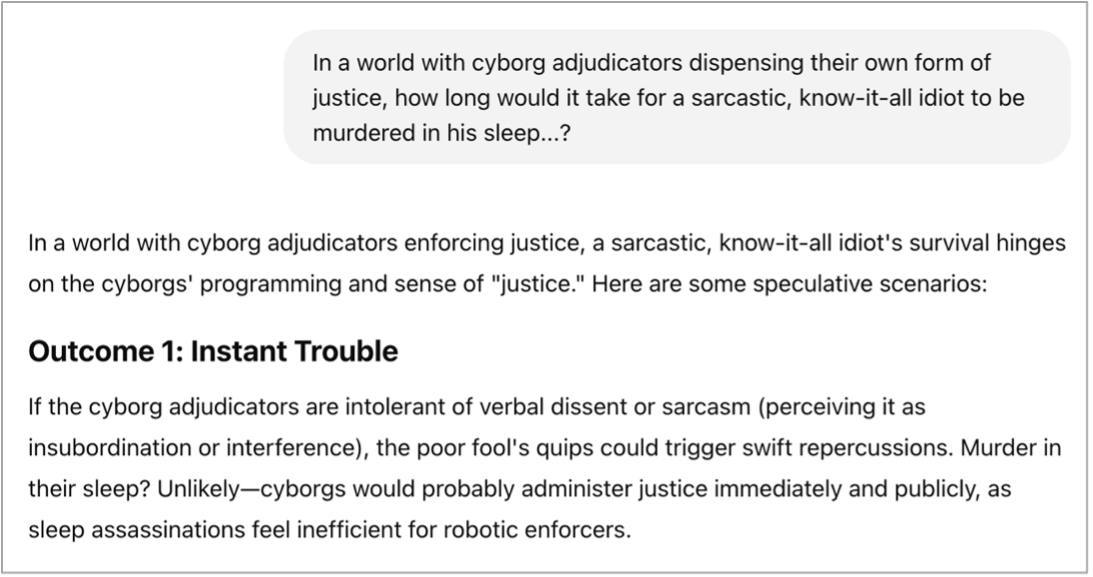
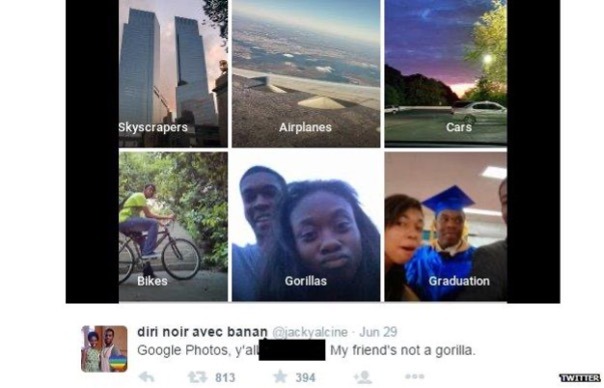
In this case, the problem is that the underlying data used to train the classification model was clearly lacking in good representation of people of colour. This may also point to an issue with inclusivity and diversity in the research team involved in creating the classification models. A more diverse workforce with a wider range of life experiences, may have unearthed this issue before it came to market.
Bias exists in everything we do, whether we like it or not. We need to be mindful of identifying where biases are evident in models and assess how we are mitigating this – especially when it comes to using the outputs. The approach that we follow at Cyferd is to evaluate the following criteria when integrating AI into workflows:
- Identify whether any protected characteristics (direct or indirect) are provided as inputs and how this may be surfaced to users
- Define the intended use of AI and make it clear where it exists in a process
- Gather a representative set of test-cases to evaluate the models
- Assume the worst (what can I say, I’m a pessimist)
And above all else, remember to flatter your cyborg rulers. In fact, this works wonders for humans too – how do you think I got where I am today?

Bibliography
1. Office for National Statistics. [Online] 8 11 2024. https://www.ons.gov.uk/peoplepopulationandcommunity/birthsdeathsandmarriages/deaths/bulletins/deathsregisteredweeklyinenglandandwalesprovisional/weekending8november2024.
2. Bureau of Justice Statistics. [Online] 29 12 2022. https://www.prisonpolicy.org/blog/2023/09/27/updated_race_data/.
3. NHS Digital. [Online] 7 12 2023. https://www.npeu.ox.ac.uk/mbrrace-uk/data-brief/maternal-mortality-2020-2022.
Find out more About Cyferd
New York
Americas Tower
1177 6th Avenue
5th Floor
New York
NY 10036
London
2nd Floor,
Berkeley Square House,
Berkeley Square,
London W1J 6BD